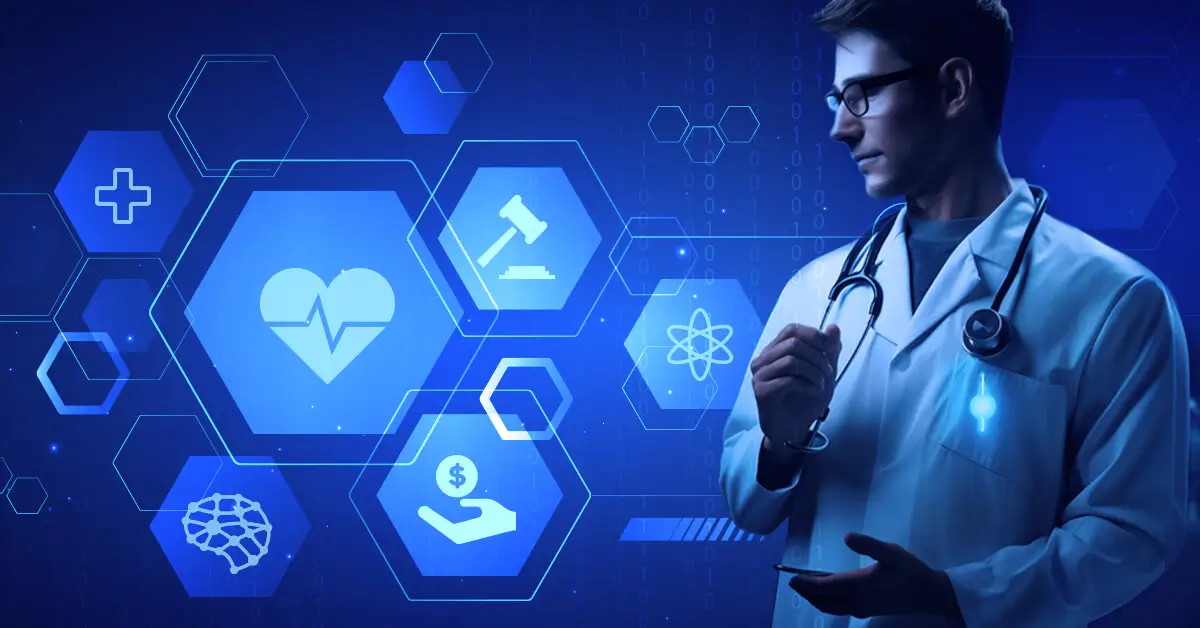
Imagine a tool that can analyze vast amounts of medical data, uncover hidden patterns, and assist healthcare professionals in making accurate diagnoses and treatment decisions without ethical problems. Enter Medical Language Models (LLMs), the cutting-edge technology revolutionizing the healthcare landscape. But with their increasing influence, it’s crucial to delve into the ethical considerations that accompany their use in medical settings.
What exactly are Medical LLMs, and why are they causing such a stir in the healthcare industry? These advanced AI models harness the power of Natural Language Processing (NLP) to comprehend and extract invaluable insights from a multitude of medical data sources. From electronic health records to clinical guidelines and research papers, Medical LLMs have the potential to enhance disease diagnosis, treatment planning, and clinical decision-making.
But as these models become more prevalent, ethical concerns come to the forefront. How do we balance the benefits of Medical LLMs with the protection of patient privacy and data security? Can we address potential biases that may impact the fairness and accuracy of diagnoses? And what about the vital doctor-patient relationship—how might it be affected by the integration of these powerful tools?
In this article, we will explore the significance of Medical LLMs in healthcare and shed light on the ethical implications associated with their use.
The Benefits of Medical LLMs in Healthcare
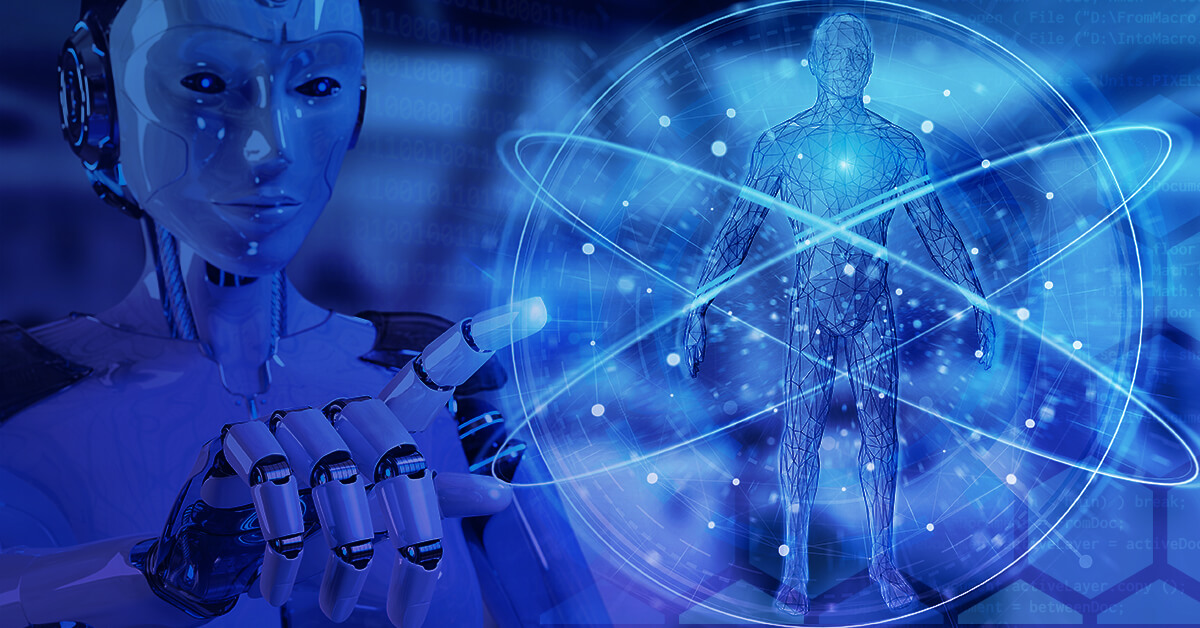
Medical LLMs, with their remarkable capabilities, have the potential to revolutionize healthcare practices and pave the way for improved patient outcomes. Let’s delve into the potential benefits these models bring to various healthcare applications, emphasizing the enhanced accuracy, efficiency, and diagnostic capabilities they offer.
- Improved Accuracy with Healthcare LLMs: Medical LLMs can process vast amounts of medical data and extract meaningful insights, aiding healthcare professionals in making more accurate diagnoses. By analyzing patient symptoms, medical history, and clinical guidelines, these models can assist in identifying complex patterns and rare diseases that might otherwise be challenging to detect. Their ability to incorporate up-to-date medical literature and research findings ensures that healthcare providers have access to the latest information, enhancing the accuracy of their diagnoses.
- Enhanced Efficiency of Healthcare LLMs: The efficient processing power of Medical LLMs can significantly streamline healthcare workflows. These models can quickly analyze patient data, prioritize cases based on severity, and provide relevant information to healthcare professionals, enabling them to make informed decisions promptly. This expeditious analysis and information retrieval save valuable time, allowing healthcare providers to allocate more attention to individual patients and deliver personalized care.
- Diagnostic Capabilities of Healthcare LLMs: Medical LLMs possess a remarkable ability to analyze complex medical data and provide comprehensive insights. By considering a multitude of factors, including patient symptoms, medical history, genetic information, and medical literature, these models can generate differential diagnoses and recommend appropriate diagnostic tests. This supports healthcare professionals in identifying potential conditions more efficiently and aids in the early detection of diseases, leading to timely interventions and improved patient outcomes.
- Tailored Treatment Plans using Healthcare LLMs: With their vast knowledge base, Medical LLMs can contribute to personalized and precise treatment planning. By analyzing patient-specific factors such as genetic information, biomarkers, and medical history, these large language models can provide insights into the most effective treatment options. This assists healthcare providers in tailoring treatment plans to individual patients, optimizing therapeutic outcomes and minimizing potential adverse effects.
Ethical Concerns and Challenges in the use of Medical LLMs
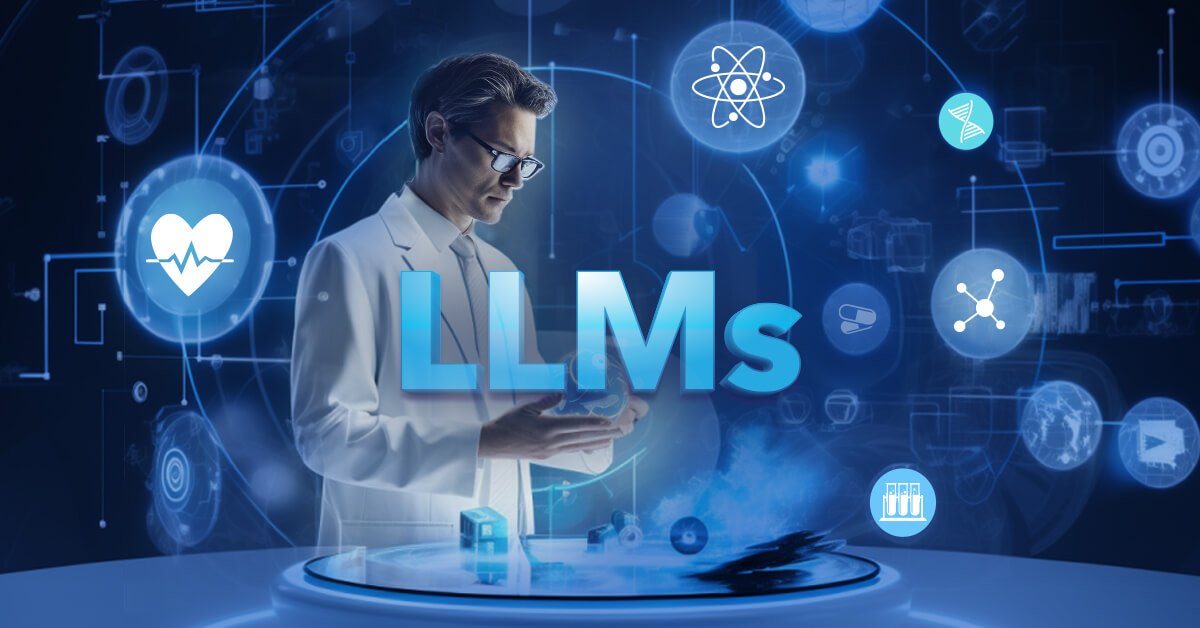
Ethical considerations play a significant role in the adoption and implementation of Medical LLMs in healthcare. While these models offer promising advancements in disease diagnosis, treatment planning, and patient care, it is essential to address the ethical concerns and challenges that arise in their usage.
1. Privacy and Data Security in the use of Healthcare LLMs
As Medical LLMs become increasingly integrated into healthcare systems, concerns about patient data privacy and security are of paramount importance. These advanced models rely on extensive patient data, including medical records, genetic information, and diagnostic imaging. While the potential benefits of utilizing this data are immense, it is crucial to address the associated risks and prioritize robust data protection measures.
One primary concern is the potential unauthorized access to patient information. Medical LLMs require access to large datasets to effectively train and optimize their performance. However, this necessitates strict protocols to ensure that sensitive patient data remains secure and confidential. Unauthorized access or breaches in data security can lead to privacy violations, identity theft, or misuse of personal health information.
To mitigate these risks, healthcare organizations must implement stringent data protection measures. This includes utilizing encryption techniques, adopting secure data storage systems, and implementing access controls to limit data access to authorized personnel only. Additionally, establishing comprehensive data governance policies and adhering to relevant privacy regulations, such as HIPAA (Health Insurance Portability and Accountability Act), ensures that patient data remains protected throughout its lifecycle.
2. Bias and Fairness in the use of Healthcare LLMs
Bias in Medical LLMs is an ethical concern that deserves careful attention. These models heavily rely on large datasets for training, which may inadvertently reflect biases present in the data. The presence of bias can lead to unequal healthcare outcomes and perpetuate disparities in patient care.
It is crucial to explore the issue of bias in Medical LLMs and understand its potential impact on healthcare outcomes. Biases can manifest in various ways, such as racial or ethnic disparities in diagnosis, treatment recommendations, or access to care. Such biases can exacerbate existing healthcare inequities and hinder the goal of providing fair and equal treatment for all patients.
Addressing bias and ensuring fairness in model development and deployment is of paramount importance. Healthcare organizations and researchers must prioritize the following considerations:
- Diverse and Representative Training Data: To mitigate bias, it is essential to ensure that training data used for Medical LLMs is diverse and representative of the patient population. Including data from different demographics, socioeconomic backgrounds, and geographic locations can help reduce biases and promote fairness in healthcare outcomes.
- Rigorous Evaluation and Validation: Rigorous evaluation and validation of Medical LLMs are crucial to identify and rectify any biases that may be present. This includes testing the models across diverse patient populations and evaluating their performance on different metrics to ensure fair and equitable results.
- Transparent and Explainable Models: Enhancing transparency and explainability of Medical LLMs can help identify and address biases. Providing clear explanations of how the models arrive at their predictions enables healthcare professionals to understand the underlying factors and assess any potential biases that may arise.
- Continuous Monitoring and Bias Mitigation: Healthcare organizations should establish mechanisms for continuous monitoring of Medical LLMs to detect and mitigate biases. Regular audits and reviews of model performance can help identify and rectify any biases that may emerge over time.
3. Explainability and Transparency in the use of Healthcare LLMs
Explainability and transparency are critical ethical concerns when it comes to Medical LLMs. These models often operate as black boxes, making it challenging to interpret and understand their decision-making process. The lack of transparency can hinder trust and acceptance among healthcare professionals and patients, raising concerns about the reliability and accountability of these models.
Interpreting the decisions made by Medical LLMs poses a significant challenge. Unlike traditional medical decision-making, where clinicians can explain their reasoning based on medical knowledge and experience, the inner workings of LLMs may not be readily apparent. Understanding how and why a particular diagnosis or treatment recommendation is made becomes essential in gaining confidence in these models.
Transparency and explainability play a vital role in gaining trust in Medical LLMs. When healthcare professionals and patients can comprehend the factors considered by the model, they are more likely to trust its recommendations and be willing to incorporate them into clinical decision-making.
To address the challenge of explainability and transparency, several approaches can be taken:
- Interpretable Models: Developing interpretable versions of Medical LLMs can provide insights into the decision-making process. Techniques such as attention mechanisms or feature importance analysis can help highlight the key factors influencing the model’s predictions.
- Rule-based Explanations: Incorporating rule-based explanations can enhance the interpretability of Medical LLMs. By providing a set of rules or guidelines used by the model to arrive at its recommendations, healthcare professionals can better understand and validate the reasoning behind the predictions.
- Documentation and Reporting: Transparent reporting of the model’s development, including data sources, pre-processing steps, and model architecture, can enhance transparency. This documentation enables peer review and scrutiny, fostering trust and accountability.
- Patient Engagement and Education: Educating patients about the role of Medical LLMs in their care and providing them with understandable explanations can help foster trust. Patient engagement in decision-making processes empowers them to ask questions and be active participants in their healthcare journey.
4. Doctor-Patient Relationship and Autonomy in the use of Healthcare LLMs
The advent of Medical LLMs has raised concerns about the impact on the doctor-patient relationship and patient autonomy. While these models offer valuable insights and support in healthcare decision-making, there is a risk of over-reliance on LLMs, potentially diminishing the human touch that is fundamental to the doctor-patient relationship.
The doctor-patient relationship is built on trust, empathy, and effective communication. It is essential for healthcare professionals to maintain open lines of communication with their patients, ensuring that their concerns, values, and preferences are considered in the decision-making process. However, the growing influence of Medical LLMs raises questions about the extent to which patient autonomy is respected.
One concern is the potential for healthcare professionals to become overly reliant on LLMs, leading to a diminished role in decision-making. While LLMs can provide valuable recommendations based on vast amounts of data, it is crucial to remember that they are tools and should not replace the expertise and judgment of healthcare professionals. Patients may feel marginalized if their preferences and values are not given due consideration when solely relying on LLM-generated recommendations.
Another aspect is the potential loss of the human touch in healthcare. LLMs excel at analyzing data and providing objective insights, but they may struggle to capture the nuances and contextual factors that are essential in personalized patient care. The doctor-patient relationship is built on empathy, understanding, and individualized attention. Over-reliance on LLMs without maintaining a human connection can lead to a loss of trust and patient satisfaction.
To address these concerns, it is crucial for healthcare professionals to strike a balance between incorporating the insights from Medical LLMs and maintaining the human touch in healthcare interactions. LLMs should be used as decision-support tools, empowering healthcare professionals to make informed decisions in collaboration with their patients.
Open and transparent communication is essential in navigating the impact of LLMs on the doctor-patient relationship. Healthcare professionals should explain the role of LLMs, their limitations, and the importance of considering patient preferences and values. Engaging patients in shared decision-making and actively involving them in the treatment planning process can help preserve patient autonomy and strengthen the doctor-patient relationship.
Mitigating Ethical Concerns in the use of Medical LLMs
Addressing the ethical implications of Medical LLMs requires proactive strategies and initiatives to ensure their responsible development and deployment in healthcare. By fostering interdisciplinary collaboration, involving stakeholders, and implementing ongoing evaluation, we can work towards mitigating ethical concerns associated with these advanced models.
Interdisciplinary Collaboration
Collaboration among professionals from various fields, including medicine, data science, ethics, and law, is essential to comprehensively address the ethical considerations of Medical LLMs. This collaboration enables a broader perspective on the potential impacts and challenges, ensuring that diverse viewpoints are considered in decision-making processes. By bringing together expertise from different disciplines, we can develop guidelines and frameworks that promote ethical practices and responsible use of LLMs.
Stakeholder Involvement
Engaging stakeholders, including healthcare providers, patients, policymakers, and industry representatives, is crucial in shaping the ethical landscape of Medical LLMs. Stakeholders have unique perspectives and insights that can help identify and address ethical concerns. By involving them in the development and implementation of guidelines, policies, and standards, we can ensure that the interests and values of all parties are considered. This inclusive approach fosters transparency, accountability, and shared responsibility.
Ongoing Evaluation
Continuous evaluation of Medical LLMs is essential to monitor their performance, identify potential biases, and address emerging ethical challenges. Regular assessment of these models allows us to understand their strengths and limitations, ensuring that they align with ethical standards. Evaluation should include rigorous testing, validation, and peer review to promote transparency, reliability, and fairness. Additionally, mechanisms should be in place to collect feedback from healthcare professionals, patients, and other stakeholders, incorporating their perspectives into the evaluation process.
Ethics Committees and Guidelines
Establishing ethics committees or review boards dedicated to Medical LLMs can provide guidance, oversight, and accountability. These committees can review the ethical implications of LLM projects, assess potential risks, and offer recommendations for best practices. Developing comprehensive guidelines and ethical frameworks specific to the use of LLMs in healthcare can help standardize their deployment, ensuring adherence to ethical principles and patient-centered care.
Conclusion
The growing role of Medical LLMs in healthcare presents both exciting possibilities and ethical challenges. We have explored the key ethical concerns associated with these models, including privacy and data security, bias and fairness, explainability and transparency, and the impact on the doctor-patient relationship and patient autonomy. While Medical LLMs offer significant advancements in disease diagnosis, treatment planning, and medical research, it is crucial to approach their implementation with a balanced perspective.
Strategies to mitigate ethical concerns include interdisciplinary collaboration, stakeholder involvement, ongoing evaluation, transparency, and the establishment of ethics committees and guidelines. By embracing these approaches, we can ensure that Medical LLMs are developed and deployed responsibly, upholding ethical standards and protecting patient rights.
In the realm of Medical LLMs, John Snow Labs has made notable contributions in utilizing these models for disease diagnosis and treatment. Their commitment to ethical practices and innovation has led to advancements that enhance patient care and improve healthcare outcomes. As we move forward, it is essential for healthcare organizations and professionals to actively engage with ethical considerations, fostering an environment that maximizes the benefits of Medical LLMs while addressing the associated challenges.
We invite healthcare professionals, researchers, policymakers, and stakeholders to join in the ongoing dialogue and collaborate in shaping the ethical landscape of Medical LLMs. By working together, we can ensure that these models are deployed in a manner that prioritizes patient well-being, respects privacy, promotes fairness, and upholds the values of healthcare ethics.
Take action today by exploring the possibilities of Medical LLMs and contributing to the development of ethical guidelines and practices. Together, let us embrace the potential of these models while upholding the highest standards of ethical conduct in healthcare.
FAQs about the Ethical Implications of Medical LLMs in Healthcare
Below are the frequently asked questions about the ethical implications of Medical LLMs in healthcare.
1. What are Medical LLMs, and how do they impact healthcare?
Medical LLMs, or Healthcare LLMs, are advanced artificial intelligence models specifically designed for healthcare applications. They analyze medical data, make predictions, and provide insights for disease diagnosis, treatment planning, and medical research. Their impact on healthcare is significant, offering improved accuracy, efficiency, and diagnostic capabilities.
2. What are the challenges for large language models in healthcare?
While large language models (LLMs) hold immense potential in healthcare, they also face certain challenges. One of the significant challenges is the need for extensive training on diverse and representative healthcare data to ensure accurate and reliable outputs. Additionally, addressing biases in healthcare LLMs and ensuring fairness in their predictions is a critical challenge. Another challenge is the requirement for explainability and transparency in the decision-making process of medical LLMs, enabling healthcare professionals and patients to understand and trust their outputs. Lastly, privacy and data security concerns must be effectively managed to protect sensitive patient information. Overcoming these challenges will contribute to the responsible and ethical use of medical LLMs in healthcare.
3. How can privacy and data security be safeguarded when using Medical LLMs?
Protecting patient data privacy and security is crucial. Robust data protection measures, such as encryption, secure storage, and strict access controls, should be implemented. Additionally, obtaining informed consent from patients and adhering to relevant data protection regulations are vital considerations in the use of healthcare LLMs.
4. How can biases in Medical LLMs be addressed?
Addressing biases in Medical LLMs requires a multi-faceted approach. It involves diverse and representative training data, rigorous validation processes, and continuous monitoring to identify and rectify biases. Collaboration with experts from various disciplines can help ensure fairness and minimize bias in these models.
5. What healthcare tasks can be automated with Medical LLMs?
Medical Large language models (LLMs) have the potential to automate various healthcare tasks, improving efficiency and accuracy. Some tasks that can be automated with healthcare LLMs include clinical documentation, such as generating reports and summarizing patient records using medical generative AI functions. Medical LLMs can also assist in natural language processing (NLP) tasks, such as extracting relevant information from medical literature and clinical notes. Additionally, medical LLMs can aid in disease diagnosis by analyzing symptoms, medical histories, and test results. They can also support treatment planning by providing personalized recommendations based on patient-specific data. Moreover, healthcare LLMs can be used for medical image analysis, detecting abnormalities in radiology scans. These automated tasks help healthcare professionals streamline workflows, enhance patient care, and make more informed decisions.